Understanding Bayesian Filter Spam: A Comprehensive Guide
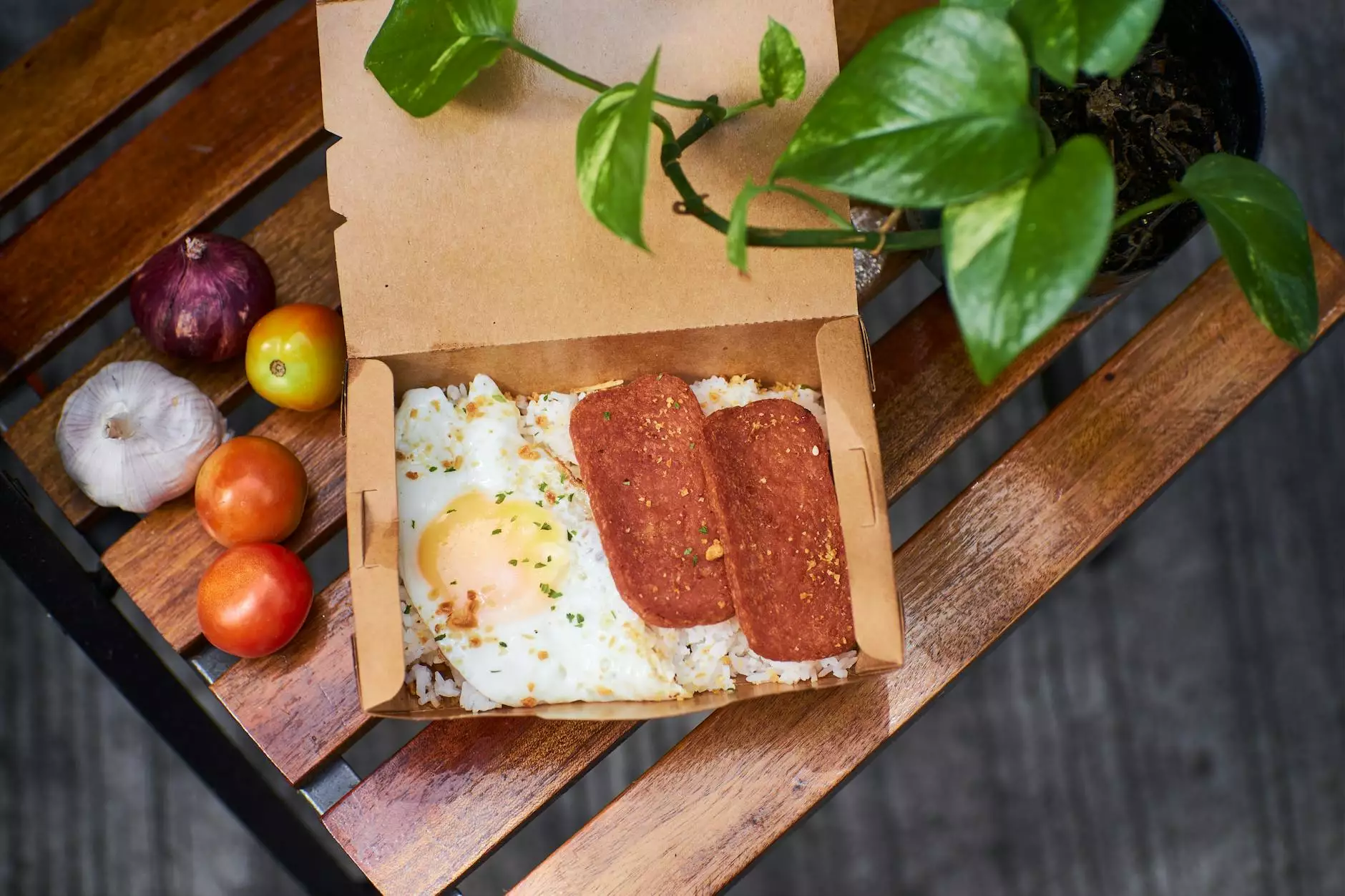
In an increasingly digital world, where communication happens through emails and online platforms, the threat of spam has become a significant concern for both individuals and businesses. The Bayesian filter spam technique has emerged as a leading method for combating these unwanted messages, providing a sophisticated approach to email security. In this article, we delve into what Bayesian filtering is, how it works, and why it is essential for IT services and businesses, such as those offered at Spambrella, in the fields of IT Services & Computer Repair and Security Systems.
What is Bayesian Filtering?
Bayesian filtering is a statistical technique used to classify or predict data based on Bayes' Theorem. It assesses the probability of an email being spam or legitimate based on the words and phrases contained in the message. The core idea is to evaluate the frequency of specific words used in both spam and non-spam emails, allowing the filter to learn and adapt over time.
How Bayesian Filter Spam Works
The effectiveness of the Bayesian filter spam mechanism lies in its ability to learn from data and make informed decisions. Here’s a breakdown of how it works:
- Data Collection: The filter collects a significant body of emails—both spam and legitimate. This corpus serves as the foundation for learning.
- Word Frequency Calculation: As the filter processes these emails, it records the frequency of individual words. For instance, common spam words like “free,” “buy now,” or “click here” are tallied separately from more neutral words found in legitimate emails.
- Probability Analysis: Using Bayes' Theorem, the filter calculates the probability of any new email being spam based on the words it contains. It adjusts its probabilities dynamically as it continues to learn from new emails.
- Decision Making: When a new email arrives, the Bayesian filter assesses the words and phrases, comparing them against its learned data. If the calculated probability exceeds a certain threshold, it classifies the email as spam.
Benefits of Using Bayesian Filter Spam
Implementing a Bayesian filter offers numerous benefits, making it a preferred choice for businesses and IT service providers focused on secure communications. Here are some key advantages:
1. High Accuracy Rate
Bayesian filters continually learn and adapt. As they are exposed to more data, their accuracy in distinguishing between spam and legitimate emails increases significantly. This means that the chances of important emails being marked as spam diminish over time.
2. Adaptability
Each organization's spam landscape can be quite different. Bayesian filters can learn from the specific types of emails that a business receives, making the filtering effective and tailored to individual needs.
3. Low Maintenance
Once trained, Bayesian filters require minimal intervention. They can operate effectively with little hand-holding, allowing IT staff to focus on other critical tasks without frequently needing to update the filter.
4. Cost-Effective
Implementing a Bayesian filter can significantly reduce the risk of spam-induced email breaches, leading to potential cost savings associated with security incidents. It’s a comprehensive approach that pays for itself over time by safeguarding valuable data.
Implementing a Bayesian Filter: Best Practices
To harness the true power of Bayesian filtering, follow these best practices:
- Build a Robust Corpus: Ensure your spam and non-spam email dataset is as comprehensive as possible to facilitate accurate training.
- Regular Updates: Continuously train and update the filter with new spam and legitimate emails to keep the filtering mechanism relevant.
- Customization: Tailor your Bayesian filtering settings to meet your specific organizational needs—adjust thresholds and sensitivity according to the business context.
- Monitor and Evaluate: Routinely assess the filter's performance, tracking false positives and negatives to fine-tune your approach over time.
Common Misconceptions About Bayesian Filtering
There are several myths surrounding Bayesian filtering that can hinder its effectiveness if misunderstood. Here are some common misconceptions:
1. Bayesian Filtering is Guaranteed to Block All Spam
While Bayesian filters are highly effective, no system is foolproof. New spam techniques constantly evolve, and it is essential to combine Bayesian filtering with other spam prevention strategies for optimal results.
2. All Spam Filters Work the Same
Different spam filters employ various technologies and algorithms. Bayesian filtering is unique in its statistical approach, which differentiates it from blacklist-based systems or heuristic filters.
The Future of Email Spam Filtering
As technology evolves, spam tactics also grow more sophisticated. The future of email spam filtering will likely see a combination of machine learning, user feedback, and advanced heuristics to combat increasingly complex spam schemes. This may include enhanced Bayesian filter spam algorithms that integrate with artificial intelligence to provide even better results.
Conclusion
The implementation of Bayesian filter spam techniques presents an effective solution for managing and mitigating the spam issue that affects businesses across sectors. By custom-tailoring spam filtering mechanisms to address the unique needs of your organization, as demonstrated by services at Spambrella, you can enhance your communication security significantly. As spam continually evolves, staying ahead with adaptive techniques like Bayesian filtering is crucial for maintaining business integrity and operational efficiency. Embrace this powerful tool and make spam a thing of the past.