The Importance of Labeled Image Datasets in Business
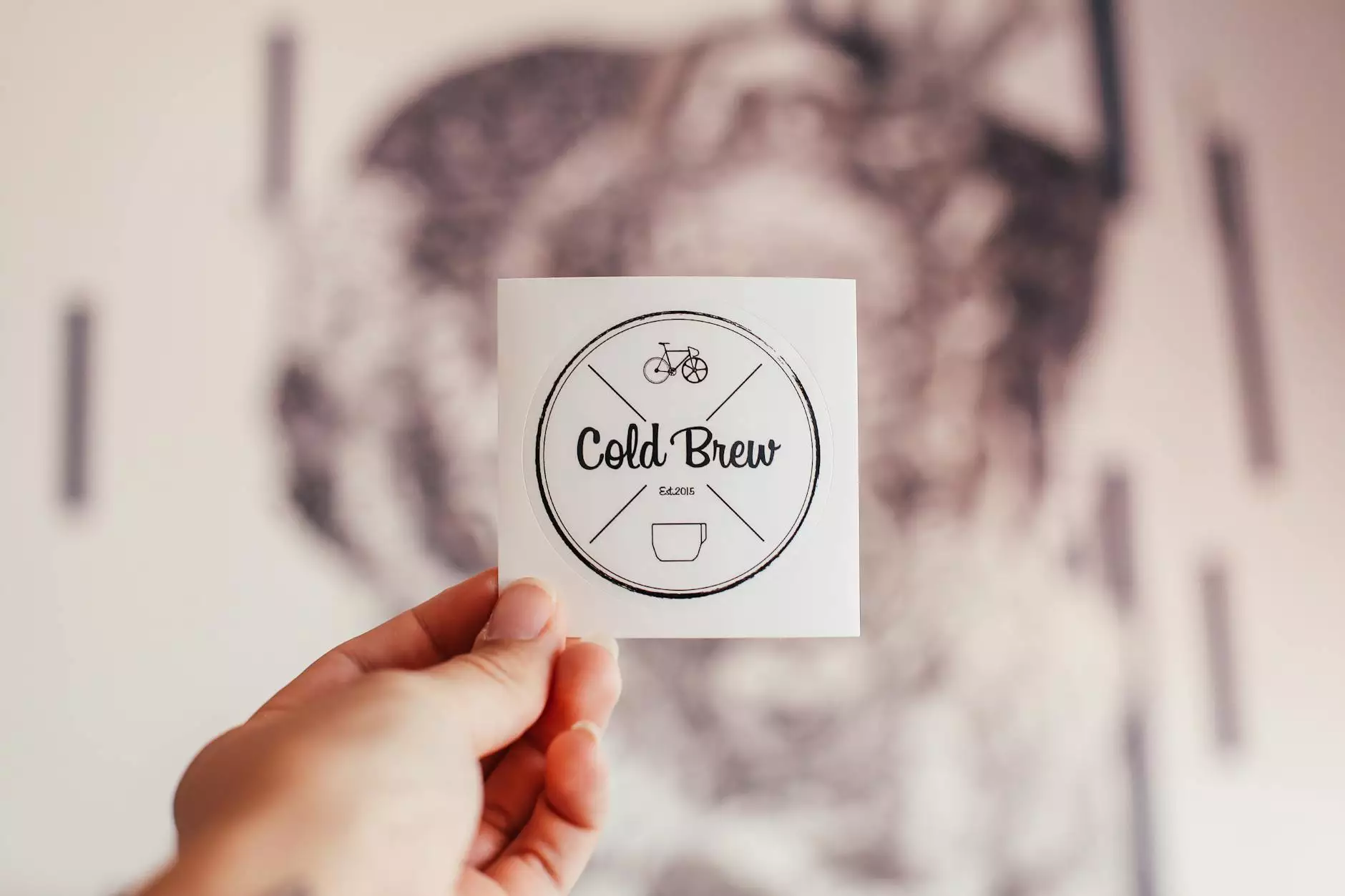
The digital landscape is rapidly changing, and the reliance on data-driven decision-making is now greater than ever. Among the wealth of data types available, labeled image datasets stand out as a transformative resource for businesses across various sectors. These datasets not only power AI and machine learning models but also streamline processes, improve efficiency, and ultimately drive profitability.
Understanding Labeled Image Datasets
At its core, a labeled image dataset consists of images that have been tagged or annotated with information that identifies objects, entities, or relevant details within the image. These labels enable machines to recognize and interpret images in a manner comparable to human perception.
For instance, in the field of computer vision, labeled image datasets are utilized to train AI models to identify and classify objects. The effectiveness of these models directly correlates with the quality and quantity of the labeled data used during training.
The Importance of Data Annotation Tools
To produce high-quality labeled image datasets, businesses require sophisticated data annotation tools. These tools facilitate the efficient annotation of images through various methods, including:
- Bounding Boxes: Drawing rectangles around objects of interest.
- Segmentation: Identifying and delineating the exact pixels of an object.
- Landmarking: Labeling specific points on an object, useful for facial recognition.
- Classification: Tagging images based on their content.
With the right tools, businesses can accelerate the annotation process, ensuring that the data used to train AI models is both extensive and accurate.
Benefits of Labeled Image Datasets
The integration of labeled image datasets into business operations comes with numerous benefits, including:
1. Improved AI and Machine Learning Models
High-quality labeled images are the backbone of effective AI training. The richer and more diverse the dataset, the better the AI model performs in real-world applications. This enhancement leads to:
- Higher accuracy in object detection and recognition tasks.
- More reliable predictions in automated processes.
2. Enhanced Customer Experience
By employing AI models trained on labeled image datasets, businesses can personalize customer experiences. This personalization can manifest through:
- Customized recommendations based on user behavior.
- Advanced customer support via chatbots that understand context through image analysis.
3. Streamlined Operations
Labeled datasets allow for the automation of various operational processes. Companies can minimize human error and increase productivity by implementing AI-powered systems that can:
- Automate inventory management through visual recognition.
- Facilitate quality control in manufacturing using visual inspections.
Keylabs.ai: Your Partner in Data Annotation
In a marketplace overflowing with data, finding a reliable partner for data annotation platforms is crucial. Keylabs.ai stands at the forefront of this industry, offering state-of-the-art solutions tailored for businesses looking to leverage the power of labeled image datasets.
Keylabs.ai provides:
- Scalable Annotation Services: Whether you require hundreds or millions of labeled images, Keylabs.ai scales its offerings to meet your specific needs.
- Expert Annotators: A team of trained professionals ensures that your data is accurately annotated, reflecting true-to-life scenarios.
- Advanced Tools: Utilizing cutting-edge technology, Keylabs.ai offers tools that simplify and expedite the annotation process.
- Quality Assurance: Every dataset undergoes rigorous quality checks to ensure it meets high standards, essential for effective AI training.
Applications of Labeled Image Datasets Across Industries
The versatility of labeled image datasets can be observed across various industries. Below are some notable applications:
1. Healthcare
In healthcare, labeled image datasets are commonly used in radiology to train AI models that can detect anomalies in medical images such as MRIs, CT scans, and X-rays. This technology supports radiologists in diagnosing diseases more quickly and accurately.
2. Automotive
The automotive industry utilizes labeled datasets to enhance the development of self-driving vehicles. By training AI systems to recognize pedestrians, traffic signs, and other vehicles, automakers can build safer autonomous navigation systems.
3. Retail
In retail, companies use labeled image datasets for inventory management and customer insights. AI-equipped cameras can monitor stock levels, identify out-of-stock items in real-time, and analyze shopper behavior by recognizing patterns in their movements and selections.
4. Security
Security systems are increasingly employing AI trained on labeled image datasets to enhance surveillance capabilities. Facial recognition technology, enabled by precise image labeling, improves security measures in public spaces and facilities.
Challenges in Creating Labeled Image Datasets
Despite the abundant opportunities offered by labeled image datasets, creating these datasets poses several challenges such as:
- Cost and Time Consumption: Manual data annotation can be a resource-intensive task, often requiring significant investment in terms of time and labor.
- Quality Control: Maintaining the quality and consistency of labels is paramount and can be difficult when managing large-scale datasets.
- Bias in Data: If labeled datasets are not diverse, AI models may develop biases, leading to skewed results in real-world applications. Ensuring diversity in datasets is essential for achieving fairness and accuracy.
Best Practices for Labeled Image Dataset Management
To navigate the challenges of labeled image datasets and maximize their effectiveness, businesses should adhere to certain best practices:
1. Employ Automated Annotation Tools
By integrating automated annotation tools, businesses can significantly reduce the time needed to prepare datasets while improving accuracy. These tools utilize machine learning algorithms to assist in initial labeling, which humans can then refine.
2. Establish Quality Protocols
Implementing stringent quality protocols is crucial when generating labeled datasets. Regular auditing and validation should take place to ensure the labels remain accurate and reliable.
3. Foster Diversity in Data
Ensure that the datasets reflect diverse scenarios that your AI model will encounter in the real world, to avoid biases and improve model performance across various demographics.
Conclusion
In conclusion, the role of labeled image datasets in business cannot be overstated. They serve as the foundation for powerful AI and machine learning applications, providing insights that drive intelligent decision-making. With the right tools and strategies, companies can harness the potential of labeled datasets to improve operational efficiency, enhance customer experiences, and remain competitive in an increasingly data-driven world.
Whether you are in healthcare, retail, automotive, or any other industry seeking to unlock the power of your images, consider partnering with Keylabs.ai. Their expertise in data annotation tools ensures you will receive high-quality labeled image datasets, tailored to meet your specific needs.